How do you Assess Image Quality?
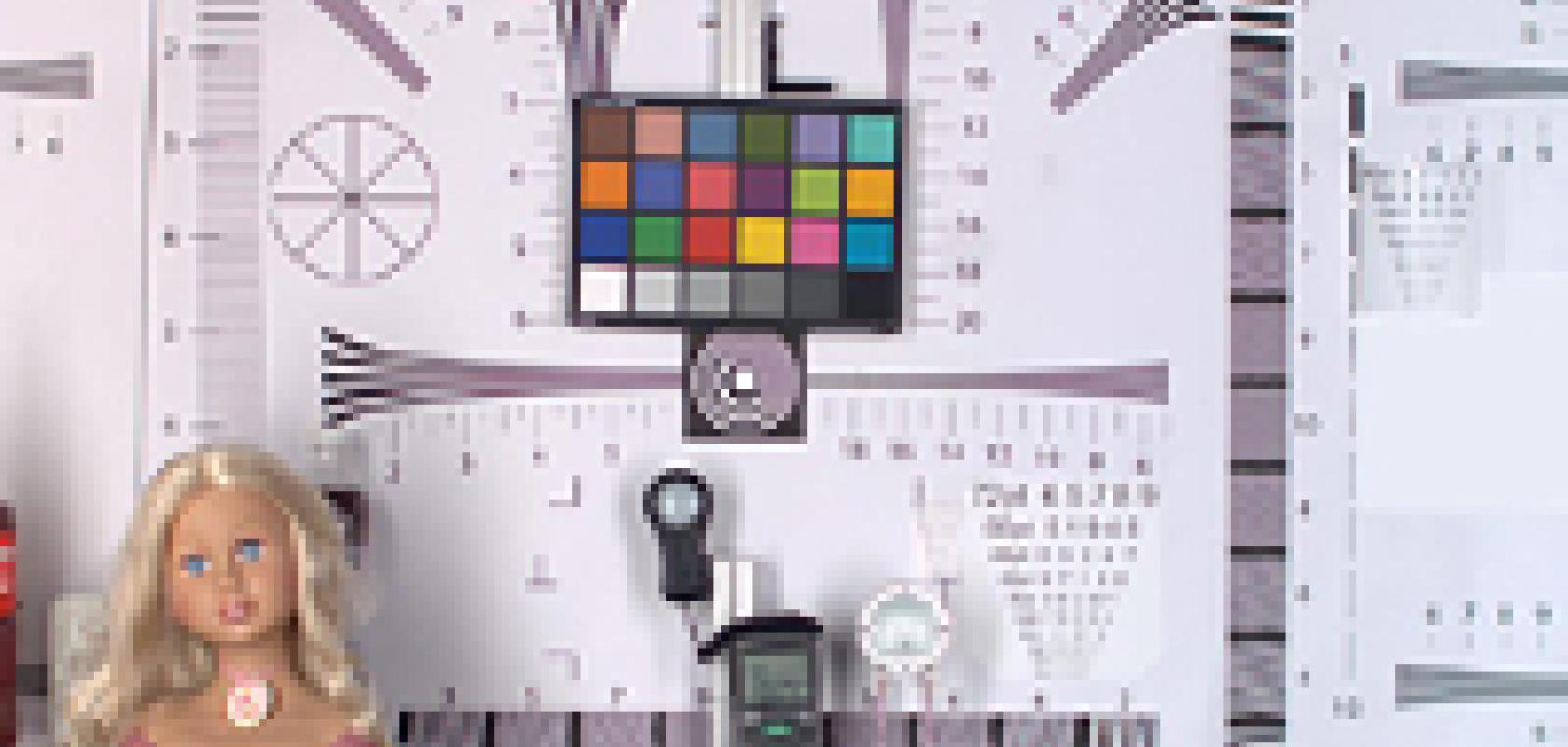
Welcome back to our series of introductory lessons on image processing! During the course of your preparations you have already made an initial pre-selection of cameras and now would like to compare the image quality of relevant models. You want to produce pictures that are sharp, high-contrast, accurately detailed, color-fast and, in particular, low in noise. There are several ways to compare these properties accurately.
Contents:
1 An Image Comparison: Quality at Second Glance
2 Improve Image with Digital Gain?
2.1 Image Noise and SNR
2.2 Light and Noise
3 Assessing Image Quality (with the Sample Use of ImageJ)
4 Assessing the Image Quality of Various Cameras
4.1 How Does one Create Comparable Conditions in Both Cases?
4.2 Two Approaches
5 Additional Factors
5.1 Quantity of Light
5.2 Sensor Size, Number of Pixels and Pixel Size
5.3 Dynamics
5.4 Resolution
5.5 Contrast and Sharpness
5.6 Color Error
6 Summary and Perspective
1. An Image Comparison: Quality at Second Glance
What is your first impression?
The left image is bright, sharp and high-contrast, while the right image is dark and somehow blurred. The outcome is seemingly evident: The left image is the better one. Or is it worth looking more closely? We want to analyze the images more precisely to find out whether our first impression passes an objective test.
2. Improve Image with Digital Gain?
The brightness of an image can be varied using digital gain. Seen superficially at first glance brighter images for the most part seem more pleasing than dark ones. Digital gain is a feature of your camera, designating the factor by which the gray values of pixels are multiplied. So, for example, all gray values of an image can be made to appear doubly bright by setting a digital gain of 6 db (factor 2). That is, you can brighten every image arbitrarily.
Inextricably linked to brightness, however, is image noise. As brightness increases, so does noise to the same extent. Since what cannot be changed when brightening through digital gain is the signal-to-noise ratio (SNR) and hence the individual property of the image.
2.1 Image noise and SNR
How does noise emerge and how does it affect the quality of an image?
An ideal camera sensor would convert a specific quantity of light to a precisely predictable quantity of electrical signals. Unfortunately, these ideal sensors do not exist since every signal processing comes with signal fluctuations, that is, noise. The noise of the electronic hardware important for our considerations is independent of the irradiated quantity of light. It is therefore already present in darkness and for this reason is called dark noise. Dark noise depends on the mounted sensor and the camera electronics but also on the temperature of components and other external interference factors. If a signal comes with noise, the noise can be reduced by software but this is very computationally intensive and is hardly possible at high frame rates. Try therefore to prevent noise from the outset. You can, for example:
- use high-grade sensors and electronic components in your camera;
- focus on a good electronic architecture in the camera assembly;
- keep the temperature of the sensor and other analog components in the camera low; and
- take precautions that prevent the interfering effect of ambient noise on the signal (e.g. using shielded cables).
The noise of the light itself is the second thing that contributes to noise. Due to statistical fluctuations, the number of photons impinging on a certain surface in a specific time varies by the square root from the number of photons. This variance is called light noise or photon shot noise. Using the sensor's quantum efficiency this noise can be transmitted to the noise of the electrons created by the sensor. The number of electrons here oscillates as well ne- (that is, the signal of the sensor) by the square root of the number of electrons √ne-.
The signal-to-noise ratio (SNR) measures the relationship between signal and noise. The SNR is expressed either as a ratio (e.g. 20 to 1) or in decibels (dB).
As described above, the camera's digital gain affects the gray value signal but, in equal proportions, also the image noise. However, the SNR remains completely unaffected by it.
=> Summary: Neither the noise nor the brightness of an image is an expressive criterion for image quality. The sole decisive property is the ratio of both factors to each other, that is, the signal-to-noise ratio. Using digital gain nothing can be changed on the SNR.
2.2 Light and Noise
The quantity of light is in a close relationship with image noise. If there is a lot of light available, it is important that the pixel processes the greatest possible number of photons, that is, a high number of electrons can be saved (high saturation capacity). If, however, little light is available, the pixel should be able to gather as many photons as possible, that is, have a large pixel surface, and convert the photons effectively into electrons (high quantum efficiency). The less light is available, the more important low dark noise becomes.
Scenario 1: Shots with a lot of light (effect of saturation capacity and dark noise):
Under shooting conditions with a lot of light, dark noise plays only a secondary role. Even when dark noise is doubled like in this example, the SNR decreases only by just under 3%. However, if the number of stored electrons is halved (for example, by a lower saturation capacity), the SNR decreases by 31%!
Scenario 2: Shots with very little light (effect of dark noise):
If only very little light is available when taking a picture, dark noise carries a lot of weight. Like in this example, the signal-to-noise ratio worsens by around 30% when dark noise doubles! Saturation capacity, however, is irrelevant in low-light conditions since the number of generated electrons lies far below the saturation capacity.
The y axis shows the SNR in decibel, bits and absolute numbers. The actual signal corresponds to a value on the x axis representing the number of absorbed photons. Saturation capacity gives the quantity of electrons which one individual pixel can absorb until it is fully saturated.
=> Summary: When there is a lot of light, the pixel's high saturation capacity is helpful and dark noise plays a minor role. In low-light conditions, however, saturation capacity is unimportant while the influence of dark noise grows.
3. Assessing Image Quality (with the Sample Use of ImageJ)
How do you assess image quality in the Machine Vision context? A practical possibility is the use of an image analytical tool such as ImageJ. This tool is a freely available program with which you can display, edit and analyze images. It, for example, adjusts contrast, changes image brightness and displays the pixel gray value in the form of a histogram.
ImageJ toolbar
To be able to assess the image quality of two cameras or of different sensors, images must first be captured under identical conditions. These are loaded into ImageJ.
You then find in the image an area that is as homogeneous as possible and place a selection rectangle in it using ImageJ.
You then generate a histogram of the image section through the key combination Ctrl+H.
See to it that when selecting the image section the mean gray value in the rectangle (called the "mean" in ImageJ) is near the saturation (>200) but that no pixel is saturated simultaneously (max <255).
The signal for our SNR calculation is therefore the mean. One must still deduct the mean from this without signal, that is, with darkened lens, to compensate for possible offset effects. This value should normally be very near zero so that it can also be disregarded when applicable. Noise in our rectangle corresponds to the standard deviation - StdDev.)
In this case, the left image has a resulting SNR of:
And for the right image:
The right image, that is, has an SNR that is better by more than a factor of 2.
This example shows how image quality can be assessed using simple tools. However, you must see to it that images are created under comparable conditions. This item will be discussed in more detail in the following section.
4. Assessing the Image Quality of Various Cameras
Following this explanation of the criteria that play a role in image assessment, the next section describes the best way to generate these images.
4.1 How does one Create Comparable Conditions in Both Cases?
Perfectly similar conditions can be created if the sensors of both cameras are of the same size. The following conditions must then be the same for both cameras:
- Object under consideration
- Illuminance
- Distance between camera and object
- Exposure time
- Image section
- Lens
- f-number of the lens
However if the sensors of both cameras are differently sized, either the distance between object and camera, the lens or the image section must definitely change.
4.2 Two Approaches
What you change and what you leave the same depends on what you want to compare. There are two possibilities here:
1. Two cameras or sensors should be compared under identical conditions as objectively as possible.
Or:
2. Two cameras should be compared under the conditions as they are also operated later in the system.
If, as described in number 1, two cameras are compared with each other, then flexibility with regard to distance or image section is allowed since the illumination of an object does not change if the distance becomes bigger or smaller or only a certain image section is tested. Details in the image can appear bigger or smaller depending on the distance from which you look at them but this does not change the decisive SNR.
If we follow the second approach and want to compare under real conditions (that means under the same conditions as in the application), distance and image section are fixed for the most part. Therefore, changes on the lens are necessary. Finally, we also no longer compare only cameras (or sensors) but rather the overall system of camera plus lens.
Ideally, for comparison purposes, use the lens that you would like to actually use later on.
5. Additional Factors
5.1 Quantity of Light
If we compare cameras with the same sensor technology and size, the quantity of light (that is, the number of photons that hit the sensor surface as soon as the shutter opens and are converted there into electrons, that is, into optical signals) can be compared easily by making sure that the same light source is used. This allows you to ensure that the images are comparable with regard to light when shooting. Create the light conditions that are also predominant later in your application.
5.2 Sensor Size, Number of Pixels and Pixel Size
The sensor is the heart and hence the most expensive component of a camera. However, its size is of course not only a question of price, but is also a decisive factor for the quantity of light that it can process. Modern industrial cameras offer a selection of various formats:
Sensor size is an important factor for image quality. Put very simply, the image quality is higher, the bigger the sensor is. However, the fact that not sensor size alone is crucial but also the number of pixels and hence pixel size is a limiting factor.
The number of pixels is measured in megapixels (MP) and it indicates how many pixels there are on the sensor surface. The higher the MP number, the higher the resolution. The often-cited guideline that, the greater the number of megapixels, the better the resulting images, is correct only to a limited extent. If there are too many pixels on the sensor surface, this leads to higher noise which, in turn, has negative effects on image quality.
Pixel size is a decisive factor in how much light a pixel can collect. A 5 µm pixel – that is, a square pixel with a 5 µm edge length – has four times more surface than a 2.5 µm pixel. It also collects four times more light and hence is clearly advantageous in poor light conditions.
Today, pixel, sizes between 3.5 µm and 6 µm offer the same output for what one needed 10 µm pixels before: a good compromise between light sensitivity and high resolution. High-resolution and favorable sensors have pixel sizes of 2.2 µm to under 1.4 µm. They offer high resolution but low light sensitivity because of their small surface.
Incidentally, this also applies to digital cameras in the consumer market. Compare a single-lens reflex camera (SLR) with a compact camera: the SLR delivers significantly better quality in low-light conditions because they are equipped with a larger sensor and hence can absorb much more light. Compact cameras are good when there is adequate light such as sunlight. As soon as you used them indoors under limited light conditions, they quickly activate the flash to capture adequate light.
5.3 Dynamics
The dynamic range of a camera describes the ratio between the strongest and weakest signal which can be identified above the noise in an image. It has an immediate effect on image quality by determining the quantity of possible gray values in the image.
What is important is the dynamic range of a camera in variable light conditions. A large dynamic range makes details in image scenes discernable, where there are bright and dark areas. This, for example, is often the case in camera applications in the traffic system. Photographs are used here, among other things, as evidence in traffic violations. The license plate of the vehicle as well as the driver are often depicted in such photographs. Depending on the lighting used, the license plate is strongly reflected but the area around the driver appears dark. So that all relevant details remain discernable despite this brightness differences, the camera should have the largest possible dynamic range. In this connection one would speak of good image quality if the details in the bright and in the dark area of an image are easily and clearly identifiable.
5.4 Resolution
When you read the specification "4 megapixels" on the data sheet of a camera, what is actually meant here is the number the pixels on the sensor surface. However, the actual resolution depends on the combination of camera, lens and distance to the object. The number of pixels (4 MP) is thereby used only as reference, what resolution the camera could produce with a lens optimally suited for the sensor and the optimal geometry to the object. In part 1 of our white paper series Fundamentals of Image Processing you will find a detailed explanation of what you should pay attention to in terms of resolution so that you obtain the best possible image quality in your shots with your camera system.
5.5 Contrast and Sharpness
The optically perceptible differences between the bright and the dark areas of an image constitute contrast. The contrast range of an image is high if it shows very bright as well as very dark areas in the image.
The contrast is the quantity of resolved details which are processed in the sensor. The better you can identify the transition from the dark to the bright strips, the higher the contrast and the sharper the image appears.
5.6 Color Error
Another criterion in image quality is the quality of color display. Differences can appear here between a camera image and the original. If the green component is missing in an image, for example, it becomes purplish. This color difference between the original and camera image is a measure of color error. It must be noted that the human eye absorbs colors clearly differently than a Machine Vision sensor. The eye is clearly more sensitive in the green range than a camera sensor.
From the purely physical standpoint, color impressions emerge due to electromagnetic waves with wavelengths between 380 nm and 780 nm. Color perception of people, however, is created by the brain and the human eye and is thereby always subjective.
To adapt the color display of a camera to the perception of the eyes and depict them realistically, the camera must carry out color correction. The required color correction depends on existing light. Basler utilizes a software tool to calibrate the camera precisely to this light. For additional information about this Basler Color Calibration Tool, the Basler Customer Service Team is available to answer your questions.
You will find more about color, color spaces, color systems, and correction of color errors in our white paper Color Calibration of Basler Cameras.
6. Summary and Perspective
We have now investigated the basic principles which play a role in the assessment of image quality. Of course there are other different tools and measures in image processing for optimizing shooting quality further. You will find soon as part of our series "Image Processing for Beginners" a detailed overview of what significance the selection of the appropriate lens has for your camera system and how you can use different camera features to your advantage. Stay tuned!